Artificial intelligence (AI) is the simulation of human intelligence processes by machines, especially computer systems.
According to a SAS Institute look at the history of AI, the term was first conceived in 1956. However, “AI has become more popular today thanks to increased data volumes, advanced algorithms, and improvement in computing power and storage.”
Artificial intelligence is a powerful tool. It automates repetitive learning and discovery through data, adds intelligence, adapts through progressive learning algorithms, analyzes more and deeper data, achieves incredible accuracy and gets the most out of data.
A novel approach to AI
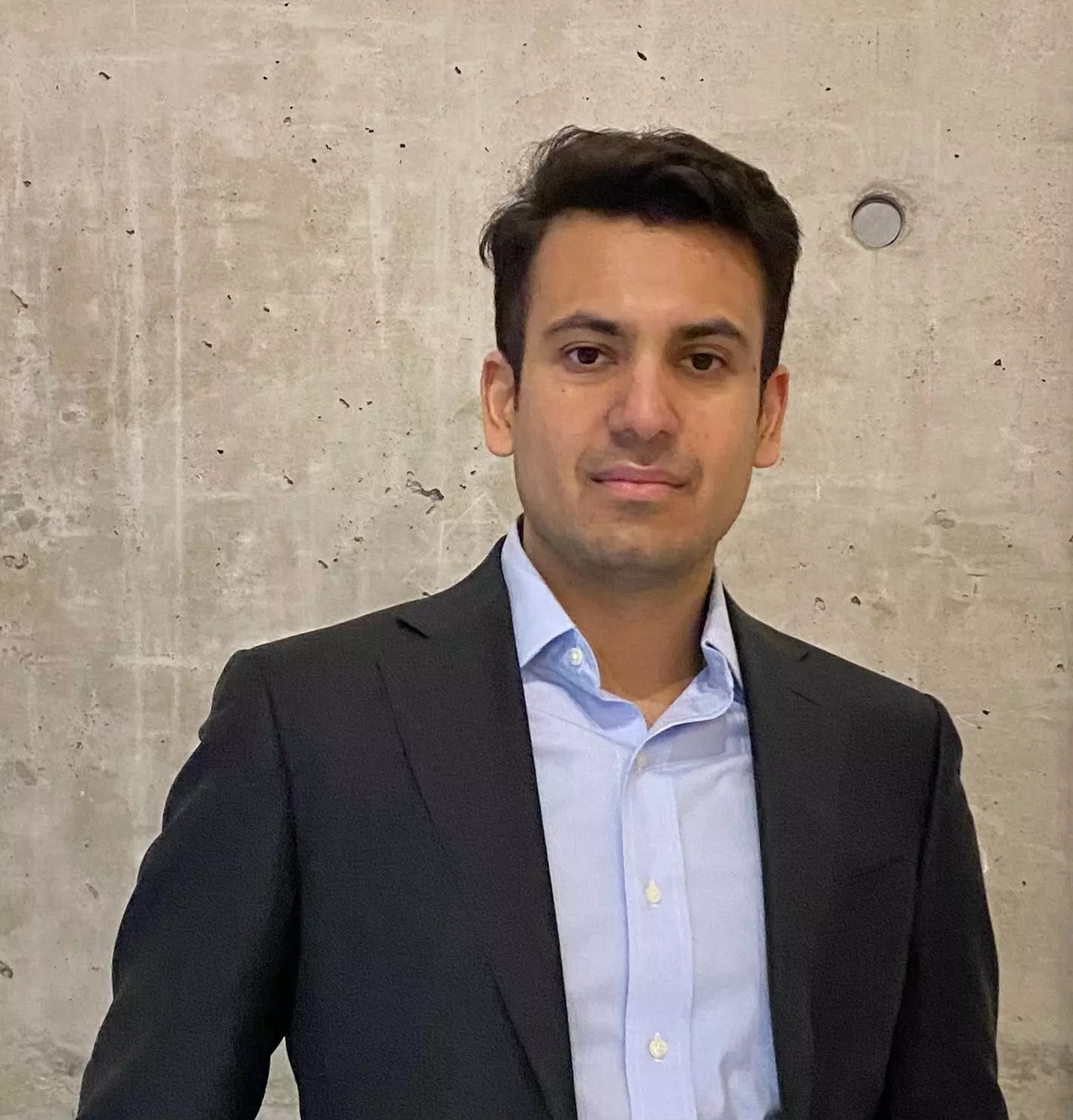
Rafid Mahmood, a Telfer assistant professor, has received a Discovery grant from the Natural Sciences and Engineering Research Council of Canada (NSERC). His AI research project proposes a novel approach to developing an operational research framework for data-centric artificial intelligence development.
Mahmood will combine machine learning (ML) methods (e.g. active learning, uncertainty estimation) used to valuate data with operational research models (e.g. optimization, queuing, simulation) to generate algorithms for decisions around managing data collection, annotation and model training.
Machine learning is a subset of artificial intelligence that allows computers to automatically learn, improve and hone their skills based on what they’re exposed to. Research on ML has studied what data can improve a model’s downstream accuracy. However, there’s been little work on how to manage the operations of the data-centric pipelines needed to field large ML projects.
Research benefits
Mahmood’s research aims to benefit those looking to implement AI systems. It could improve the success rate of AI deployment in enterprises with limited ML expertise. AI technology companies may be interested in this research to minimize annotation costs and workflow delays from having to address data limitations mid-project. Finally, emerging data collection and annotation companies may benefit from optimizing their current data management workflows.