The future is here: Analytics has transformed how financial institutions manage risks
With growing warnings of risks of a financial crisis worldwide, finding tools that help banks navigate the uncertainties of the financial world is extremely important. Since the 2008 financial crisis, banks have invested heavily on new technologies to improve their risk management strategies.
A multidisciplinary field that uses mathematics, statistics, and computer science to interpret and predict relevant patterns in recorded data, analytics has dramatically transformed how financial institutions approach risk management. A McKinsey & Company report shows that, while 15% of functions’ staff in banks worked in analytics in 2016, this number will be close to 40% by 2025.
“Analytics has become a valuable tool helping financial institutions such as banks foresee potential risks and be better prepared for the uncertainty of the financial market,” explains Telfer School of Management Professor Jonathan Li, a specialist in business analytics and financial mathematics. If banks make better predictions, they are also more likely to make the right investment decisions as economic scenarios emerge.
But analytics has limitations. Professor Li explains that one of the current challenges in using analytics to predict market behaviour and manage risks is that most models are based on a historical data and do not “look into the future”.
For example, when banks are concerned about the risk of mortgage defaults, their financial analysts sift through data of all mortgage loans during the past 10 years and look for patterns that indicate that a similar behaviour is likely to happen in the future.
“But does a model that measures the risk of loss based on past market behavior allow us to give a better picture of the risks banks will face?” asks Professor Li. As the financial market depends on a series of complex factors, there is no guarantee that history is likely to repeat itself again.
“The predictions based on historical data of market behavior give you a great starting point, but banks have to be careful when making decisions based on too many unknown factors,” explains Professor Li. When relying too much on historical data, analytical models may create a distorted picture of future market behaviour.
To address this problem, Professor Li has developed advanced mathematical models that complement the current measures of loss. These results have been accepted in Operations Research—the best journal in operations research.
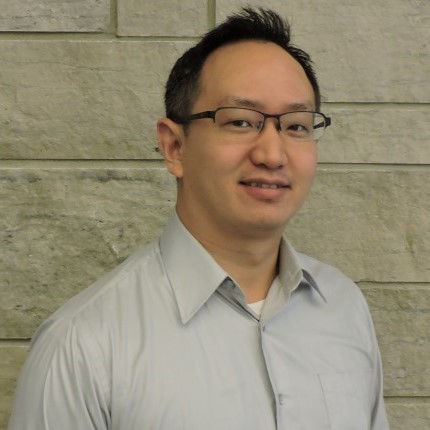
“Financial institutions should not rely solely on historical data but instead look for more systematic analytical tools to measure risk. Such complementary tools, like the ones I am proposing, will help to create risk models that well-represent what is likely to happen in the market in the future.”
Who gains?
When equipped with the best analytical tools and models, banks leverage their ability to navigate the investment market and ultimately to improve their bottom line.
According to Professor Li, apart from working with most up-to-date models and tools, banks also need to develop consistent language and practice when communicating an unfavourable scenario to regulators and clients. Oversimplifying or poorly explaining risks can lead to bad decisions.
Finding solutions that can help banks to better measure and control their loss will also benefit society in general. The decisions banks make not simply affect major investors and big corporations, but also anyone who has mutual funds, retirement funds, or an education fund for their kids.
As the average bank client doesn’t have sufficient knowledge to evaluate risks and make optimal investments, they rely on the choices that these financial institutions make. If banks are well prepared, their clients will have fewer surprises.
What to learn more about research in risk management?
If you would like to learn more about how analytics can help banks improve their risk management strategies, contact Professor Li.
Don’t miss out the 2018 Quantitative Risk Management & Financial Analytics Workshop that the Telfer School of Management is organizing in collaboration with the Department of Mathematics and Statistic on May 10th.