New Faculty Seminar Series - Rafid Mahmood
Optimizing Data Collection for Machine Learning
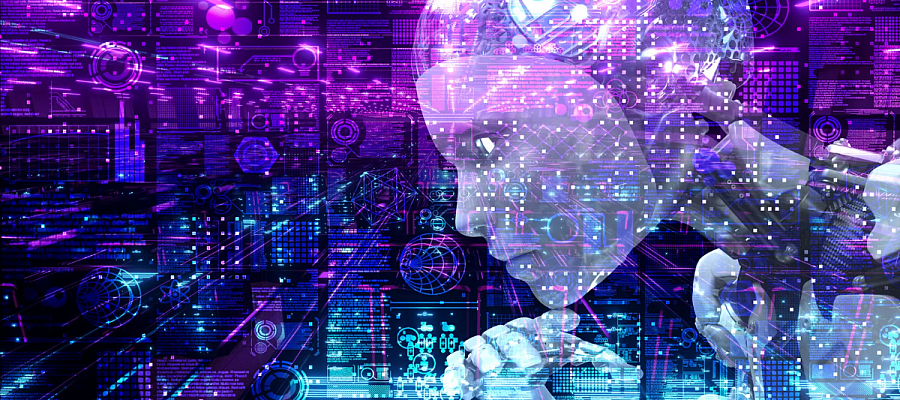
Artificial intelligence (AI) systems using deep learning are trained with massive data sets. However, there is little guidance on how much or what kind of data is needed to train these models. Over-collecting data incurs unnecessary costs, while under-collecting may delay workflows with post hoc costs. We propose a new framework to model the data collection workflow as a formal optimal data collection problem that allows designers to specify performance targets, collection costs, a time horizon, and penalties for failing to meet the targets. This formulation generalizes to tasks with multiple data vendors and permits custom analyses such as how to upgrade an existing AI model or to choose between competing collection policies. To solve our problem, we develop Learn-Optimize-Collect (LOC), which estimates and minimizes expected collection costs. Finally, we numerically compare our framework to the conventional baseline of estimating data requirements by extrapolating from neural scaling laws. We significantly reduce the risks of failing to meet performance targets on six computer vision applications, while maintaining low total collection costs.
About the Speaker
Rafid Mahmood is an Assistant Professor at the University of